The first Autonomous Tech Conference took place on Oct 21 – Nov 1, 2018 at Tel Aviv Convention Center.
Orr Danon spoke at a conference on “Deep Learning Processing Technologies for Embedded Systems”.
Abstract:
Deep learning has become the most prominent technique for image processing in recent years. Alongside its superior accuracy in many tasks and its ability to adopt through training to various use cases, deep learning presents a significant challenge to available processors. The resources required for typical Neural Networks (NNs), when applied to real life use cases such as high resolution, real time sensory data, approach the high 10s terra multiply-and-add operations per second (TMACs), let alone the associated memory bandwidth needed for the task, and pose a significant challenge to existing processors’ technology.
Current state of the art solutions for running NNs are better suited for data center environment and are inclined to trade-off efficiency for the sake of higher peak performance. Similarly, latency can be compromised to gain better performance, in which case techniques such as batch processing can be employed. In an embedded environment, power consumption and cost are typically limited by a relatively rigid envelope. Processing must be carried out on the fly, image-by-image. This presents a new set of challenges, which require a new approach for designing processors to best fit the problem domain.
In this talk, we will present the problem of image processing in a typical AV/ADAS scenario, both in terms of required resources and of compute paradigm. We will start by exploring the theoretical limits for processing efficiency, compare this with reported results from various players in the industry, and analyze some of the deficiencies which contribute to the large gap between theory and practice for different approaches. A case study analysis will follow. We shall pick a case such as rear-view camera feed for parking assist as an example. This case will be thoroughly analyzed to exemplify some of the statements previously made. This exploration will float some of the inherent system issues, performance requirements and the huge potential unlocked by a capable solution for running NNs locally. We shall conclude with guidelines for designing processing systems which are able to achieve the required efficiency for running state of the art deep learning on embedded devices.
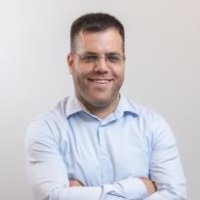
Bio:
Orr brings more than a decade of experience from the Israel Defense Forces where he led an elite Technological Team to success in some of the largest and most complex interdisciplinary projects in the Intelligence Community. Orr has been recognized with the Israel Defense Award granted by the President of Israel and the Creative Thinking Award bestowed by the Head of Military Intelligence.
Orr holds a B.Sc. in Physics and Mathematics from Hebrew University as part of the Talpiot program and an M.Sc. in Electrical Engineering (cum laude) from Tel-Aviv University.
For Orr Danon’s presentation click here
Legal Disclaimer:
You understand that when using the Site you may be exposed to content from a variety of sources, and that SagivTech is not responsible for the accuracy, usefulness, safety or intellectual property rights of, or relating to, such content and that such content does not express SagivTech’s opinion or endorsement of any subject matter and should not be relied upon as such. SagivTech and its affiliates accept no responsibility for any consequences whatsoever arising from use of such content. You acknowledge that any use of the content is at your own risk.
Leave A Comment