The annual Israel Machine Vision Conference (IMVC) took place on March 28, 2017 at David InterContinental Tel Aviv.
Eddie Smolyansky spoke at a conference on “Physical to AR – Spatiotemporal Object Representation using CNN Video Segmentation”.
Abstract:
The task of category independent foreground segmentation in images is challenging for a machine learning system, because it needs to learn the general concept of an object, even for object categories that it hasn’t seen during training. In the case of foreground segmentation in videos, the problem is compounded by the fact that the object as well as the background change appearance throughout the video. We propose a method for learning the general concept of object appearance in videos, based on deep neural networks. Apart from learning the object appearance for each frame, our system learns the temporal changes between frames in a video, which represent the object motion, and thus leverages the temporal information available in videos. By learning a category-independent object segmentation, we are able to perform unsupervised video object segmentation. In addition, in the case of semi-supervised video segmentation (where one frame from the video is annotated) we further train our system to recognize a specific object which appears in the video. In both scenarios, our system compares favorably against the state of the art.
Furthermore, we demonstrate a novel use case for video object segmentation, by implementing a mobile application where a user captures a video of an object, and our system is able to segment the object and display it in an AR setting.
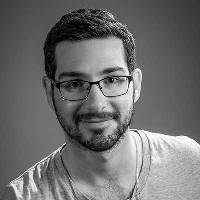
Bio:
Eddie joined Visualead as an accomplished computer vision engineer and quickly grew to lead Visualead’s Research vision and efforts. Always working on the Next Big Thing, the highly academic research team is responsible for the company’s innovation and next generation of disruptive technologies. Prior to Visualead, Eddie worked in the smartphone dual-lens company CorePhotonics.
In the IDF, he took part in the prestigious Psagot program and later served as an academic officer in the unit 8200, where his team won the Israel Defense Award (among others). He holds two B.Sc. degrees from the Technion in EE and Physics.
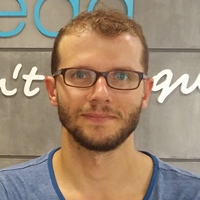
Bio:
Gilad is Visualead’s Senior Computer Vision Researcher, where he applies deep learning research on innovative scenarios. Gilad holds a B.Sc. in Electrical Engineering and Physics, and an M.Sc. in EE from Tel Aviv University.
He specialised as a computer vision researcher in VISICS lab at KUL university, where he worked on implementing advanced algorithms for action recognition from videos. Gilad published several research-papers in leading computer vision conferences on the topics of image segmentation and action recognition.
Legal Disclaimer:
You understand that when using the Site you may be exposed to content from a variety of sources, and that SagivTech is not responsible for the accuracy, usefulness, safety or intellectual property rights of, or relating to, such content and that such content does not express SagivTech’s opinion or endorsement of any subject matter and should not be relied upon as such. SagivTech and its affiliates accept no responsibility for any consequences whatsoever arising from use of such content. You acknowledge that any use of the content is at your own risk.
Leave A Comment